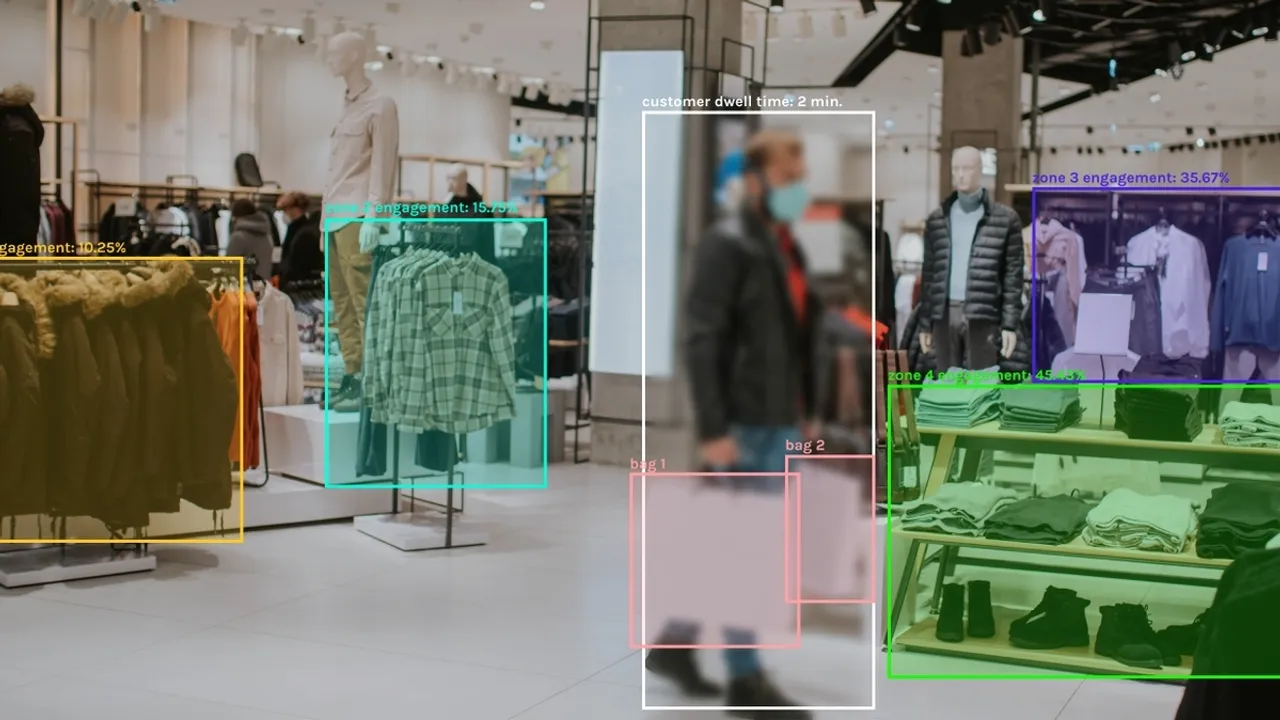
Challenge
One of the well-known clothing stores in Sri Lanka faced a significant challenge in adapting to the demands of today's dynamic economy, specifically the need for comprehensive customer information. This issue held particular significance for their business, which heavily relied on in-person interactions. The manual tracking of customer behavior proved to be a challenging and error-prone task, even with a dedicated team that was cognizant of peak shopping periods and popular hours.
Some stores found themselves grappling with limited staff, leading to concerns about allocating resources efficiently. The need for a data-driven approach became undeniable. It became imperative to optimize staff allocation and strategically deploy resources during peak hours. In this fast-paced industry, where trends change in a blink, the retail chain had big questions.
- Who typically visits the store during peak times, and what times do they usually arrive?
- Where should they focus attention, especially in stores with limited staff?
- Why were some stores busy but not making many sales?
Obtaining precise customer data, including visitor counts, mapping the busiest retail sections, and understanding consumer behavior, remained a significant challenge. The store's capacity to optimize operations and enhance revenue was hindered by the lack of accurate data. The absence of precise information made it challenging to strategically position products, efficiently manage staffing, and launch promotions that resonated with customers, ultimately impacting the overall shopping experience. Recognizing the imperative for improved data collection and analysis capabilities, the business aimed to mitigate the risk of overlooking crucial insights that could bolster sales and foster client loyalty. In today's competitive landscape, successful utilization of customer information for data-driven decision-making in retail poses an ongoing challenge.
Solution
When confronted with various obstacles, a resolute commitment to exploring novel solutions ensued. This led to the remarkable innovation of SenzView, a groundbreaking real-time customer behavior analysis tool by SenzMate. SenzView, an AI application, revolutionized the retail landscape, empowering staff to make informed, strategic decisions. The tool facilitated the detection of incoming customers, counting them every two minutes and sending pertinent data to a cloud platform. With SenzView, the organization was empowered to make decisions that not only bolstered their financial performance but also elevated the shopping experience for their cherished customers. It proved to be a transformative force, heralding success in the challenging retail landscape.
Our solution offers businesses an in-depth understanding of their consumer dynamics by seamlessly integrating into the retail scene through the use of machine learning models, integrated IoT Edge devices, and an effective software platform.
The below figure illustrates the process of how the customer behavior is analyzed.
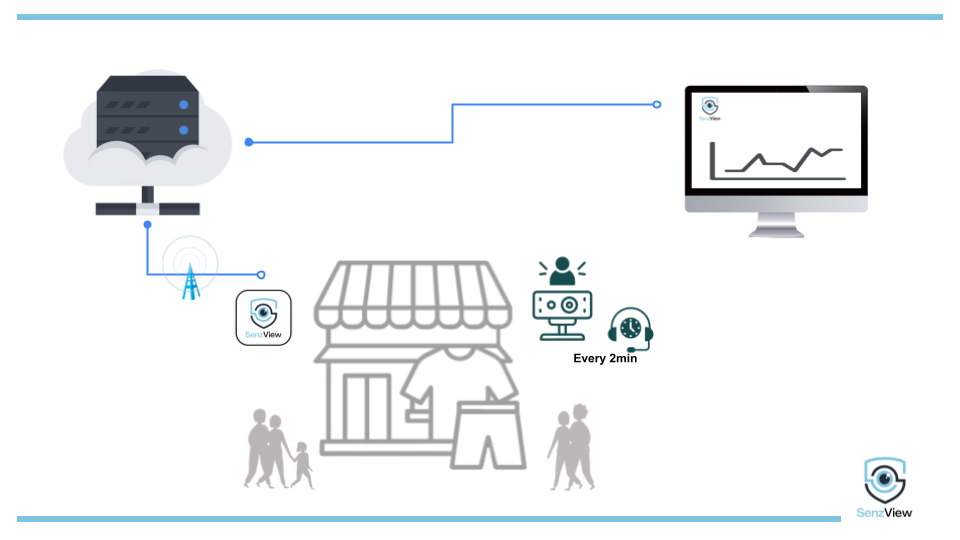
Idea Stage
As we embarked on the journey to create SenzView, our first consideration was the technology foundation that would capture the essence of customer behavior in retail spaces. Sensors seemed like an obvious choice initially, but a critical drawback surfaced the potential to miscount when multiple people crossed simultaneously. This led us to explore an alternative: cameras, which offer a more nuanced and accurate way to gather information.
The next pivotal decision revolved around whether to opt for existing cameras or invest in new ones. Evaluating the pros and cons, we leaned towards utilizing existing cameras with a touch of integration. This not only proved cost-effective but also ensured a smoother transition for businesses already equipped with surveillance systems.
In the ideal stage of our project, below is how we came up with our technologies to build up this successful customer behavior analysis platform.
- We initially evaluated the Raspberry Pi device and Intel NUC, but its processing power proved insufficient. We then turned to the Jetson from NVIDIA.
- The heart of our model was YOLO, a robust object detection model, which was supposed to be quite large. As we cannot compromise on accuracy, we planned to use the YOLO Tiny version.
- For model optimization, efficiency on edge devices, and ensuring swift and accurate detection, we planned to use TensorRT.
- To transmit the detected data to the cloud platform, we planned to use MQTT, a protocol designed for passing messages from servers to cloud platforms.
- Importantly, we planned to utilize the same CCTV cameras already in place at the clothing store for our detection purposes.
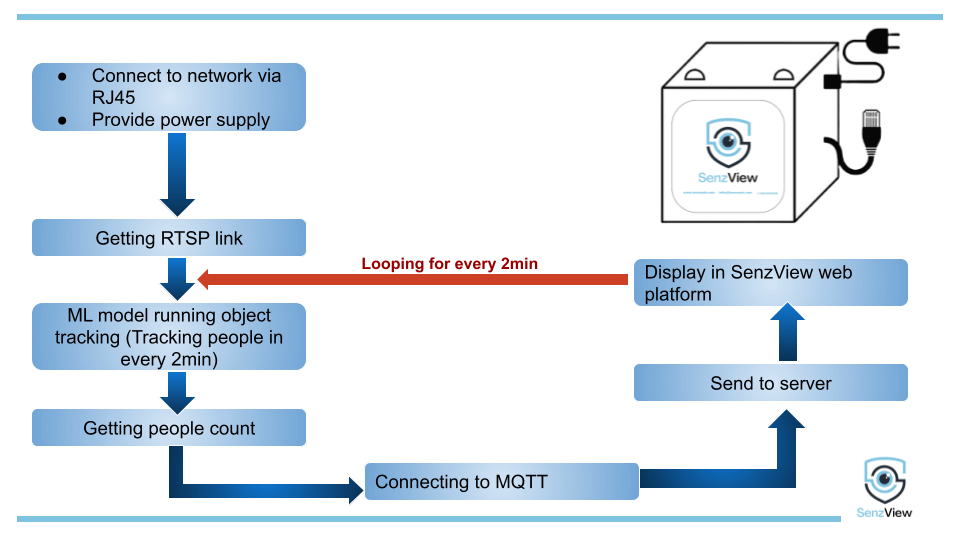
Proof Of Concept
In the pursuit of bringing SenzView to life, the proof of concept (PoC) became a crucial phase, illuminating the path toward a revolutionary customer behavior analysis solution. The central idea was to connect cameras to an AVR (Analog Video Recorder) or DVR (Digital Video Recorder) and establish a wireless link to the NVIDIA Jetson platform using the Real-Time Streaming Protocol (RTSP).
We executed our Proof of Concept, evaluating SenzView in our office setting using a single CCTV camera positioned at the entrance. We explored the potential for wireless connectivity between AVR and NVIDIA Jetson, and we assessed the precision of people identification and counting features.
Our model was designed to identify individuals moving near the entrance, considering them the same person within close time intervals of 2 minutes. To achieve this, the model temporarily stored the relevant person's features, ensuring that they were not double-counted. We implemented an imaginary line across the entrance door to determine whether a person was entering or exiting.
Challenges Uncovered:
- Accuracy: One notable challenge that emerged during the PoC was ensuring the accuracy of the data collected. While RTSP facilitated the wireless transmission of video feeds, occasional discrepancies were observed in the synchronization of data from multiple cameras. This issue prompted a meticulous examination of the data transmission process to optimize accuracy.
- Latency: Another area of concern was latency in data transmission. The real-time nature of customer behavior analysis demands minimal delay, yet our initial tests revealed instances of latency. This discovery prompted a thorough investigation into optimizing the wireless connection and data processing pipeline to mitigate delays and ensure timely insights.
Mitigation Strategies: To address these challenges, our team embarked on a refinement process. For accuracy, we fine-tuned the synchronization algorithms, ensuring that data from multiple cameras was aligned seamlessly. Additionally, efforts were directed towards optimizing the wireless connection to minimize latency, enhancing the responsiveness of SenzView.
Minimum Viable Product
As SenzView transitioned from concept to reality, our initial focus was on crafting a Minimum Viable Product (MVP) that could showcase the transformative power of our customer behavior analysis solution. Choosing one customer outlet as our testing ground, we embarked on a journey of deployment, refinement, and feedback collection during a month. During this period, we encountered several noteworthy challenges.
- Initially, we faced data loss issues when the device encountered network errors or was powered off. To mitigate this, we implemented a local data storage solution, ensuring that valuable data could be retrieved later from the database.
- We discovered that the accuracy of the model was highly influenced by factors such as camera angle and the distance between the camera and the door. Notably, when three individuals entered the store simultaneously, the model mistakenly identified them as a single person.
- The model encountered challenges in accurately identifying individuals, particularly when they were wearing traditional attire such as a burka, which is a long, flowing garment covering the entire body from head to feet. This presented difficulties in determining whether a person was entering or exiting.
- To provide more efficient support to the clothing store in case of issues, we established a cloud-based platform for device status monitoring and troubleshooting, eliminating the need for physical visits to the store.
- We encountered a time gap between the outputs from the RTSP and those from the DVR. To bridge this gap and ensure real-time outputs, we diligently worked on increasing the inference speed, successfully reducing the inference delay.
To bring our idea to life, we required a high-processing power model coupled with an object detection model known for its precision. Additionally, we addressed the issue of heat generation within the devices by implementing effective cooling solutions, such as fans, to ensure optimal performance and device longevity.
Here is the deployment process:
- Integration with AVR/DVR: Our first step involved seamlessly integrating SenzView with the customer's existing surveillance infrastructure. We connected our device to the AVR/DVR, ensuring compatibility and a smooth transition.
- Network Integration: To establish a robust connection, we seamlessly integrated our device into the customer's network. This step allowed for streamlined data flow and efficient communication between the cameras, our device, and the SenzMate Cloud.
- Camera Relocation: Focusing on one gate for our MVP, we strategically relocated cameras to optimize coverage and gather detailed insights into customer behavior. This step aimed to showcase the adaptability and effectiveness of SenzView in a real-world retail scenario.
Here is the feedback Collection:
User Experience Testing: Actively involving the customer in the testing phase, we sought feedback on the user experience. This encompassed the ease of navigating the web interface, interpreting data, and accessing relevant information.
Improvement Suggestions: Engaging in a collaborative dialogue, we gathered suggestions for improvements and additional features. This iterative process aimed to align SenzView more closely with the customer's unique requirements and expectations
Commercialization
Having validated our vision through a successful MVP, SenzView is now poised to revolutionize the retail landscape as we transition into the commercialization phase. This marks a significant milestone, signaling our commitment to deploying our advanced technology solution across multiple outlets. What was once an experimental trial has evolved into a comprehensive strategy as we go all in to seamlessly integrate our system into each outlet.
The below figure shows the SenzView Customer Behavior Analysis Dashboard that the above company got more insights to improve their business activities.
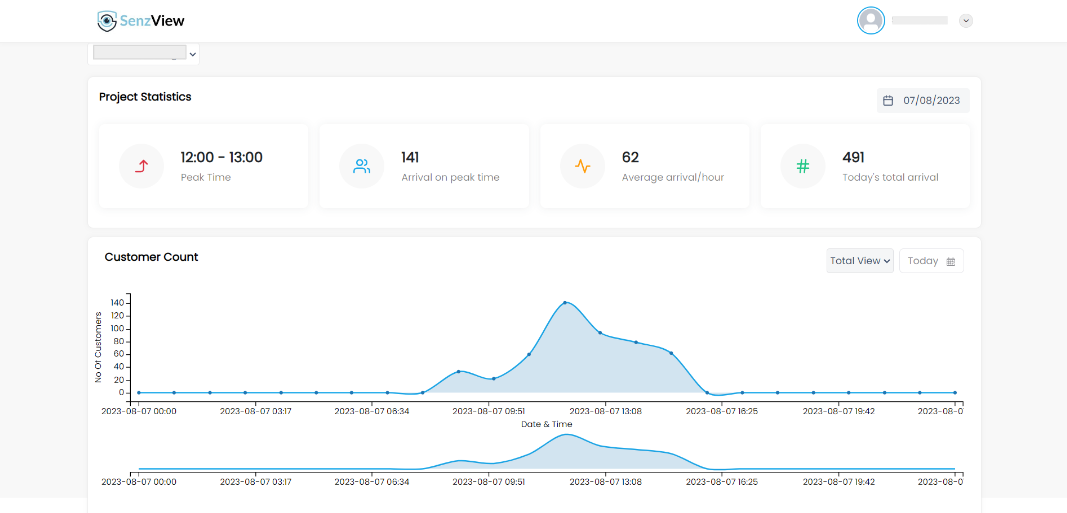
Here are the key components of the commercialization plan:
- Scalability with 10/15 Showrooms: With confidence drawn from the success of the MVP, we are set to deploy SenzView in 10/15 showrooms, ensuring a broader scope and validating the scalability of our solution across diverse retail environments.
- Enhanced Precision and Accuracy: The core functionality of SenzView remains centered around delivering accurate footfall counts and precise recognition of entries and exits. This ensures that the system continues to provide real-time insights into customer demographics, enabling businesses to make informed decisions.
- Remote Access Capabilities: Recognizing the need for flexibility and convenience, we've incorporated remote access capabilities into SenzView. This feature allows businesses to monitor and manage the system from anywhere, providing real-time updates and ensuring operational efficiency.
- Multi-Gate Functionality: Recognizing the varied layouts of retail outlets, SenzView now offers enhanced functionality for multiple gates. Whether it's a single entrance or a complex layout with several gates, our solution adapts seamlessly, offering a tailored experience to meet the unique needs of each outlet.
- Web Dashboard Refinement: Building on the success of our initial web interface, we've refined the dashboard to be even more intuitive. The addition of a layout map with a heatmap provides a visual representation of customer distribution within the outlet. This not only enhances the user experience for businesses but also facilitates quicker decision-making based on actionable insights.
- Graphical Representation of Data: To enrich the analytical capabilities of our clients, we've introduced graphical representations of key data points. The web dashboard now includes detailed graphs showcasing customer behavior trends, product popularity, and peak hours. This empowers businesses with a holistic view of their retail dynamics.
Results
SenzView has proven to be a game-changer for every outlet of the clothing store, empowering staff with the tools they need to make informed decisions and run their stores more efficiently. By providing valuable insights into customer behavior, SenzView has enabled them to enhance their operations and significantly improve the overall customer experience. This success underscores SenzView's steady commitment to delivering state-of-the-art solutions that precisely meet the evolving demands of the retail industry. As we continue to expand our presence and influence in the market, we are excited about the future possibilities and the positive impact we can bring to even more businesses. Our mission remains focused on driving innovation, efficiency, and growth for our partners, and we are eager to embark on this journey of continued success.
Written by Naduni and Hansika